How Does Artificial Intelligence Learn?
Artificial Intelligence (AI) has become an integral part of modern business. Its potential to automate processes, optimize operations for increased profitability, and support decision-making is invaluable. However, AI penetrates almost all areas of life – not just business, but also entertainment, medicine, and science. What enables AI system to have such wide applications is its ability to learn, allowing it to adapt to new situations and improve performance over time.
Like humans learn through experiences, experimentation, and absorbing new information, AI develops its skills by analyzing vast amounts of data. This analogy to the human learning process is particularly evident in the concept of neural networks, which mimic the functioning of the human brain, and in machine learning. Let’s explore how exactly AI learning works.
Basics of Artificial Intelligence
Artificial intelligence is a field of computer science focused on creating computer systems and algorithms capable of performing tasks that typically require human intelligence. AI focuses on creating algorithms and models that can analyze vast amounts of data, find patterns, and draw conclusions based on that data.
Practical applications of AI include recommendation systems on e-commerce platforms, chatbots in customer service, autonomous vehicles, business data analysis, and diagnosing diseases from medical images.
AI encompasses a wide range of technologies, including:
- Expert Systems: programs that use specialized knowledge to make decisions in narrowly defined areas.
- Natural Language Processing (NLP): technologies that enable computers to understand and generate natural language.
- Computer Vision: systems capable of recognizing and interpreting images.
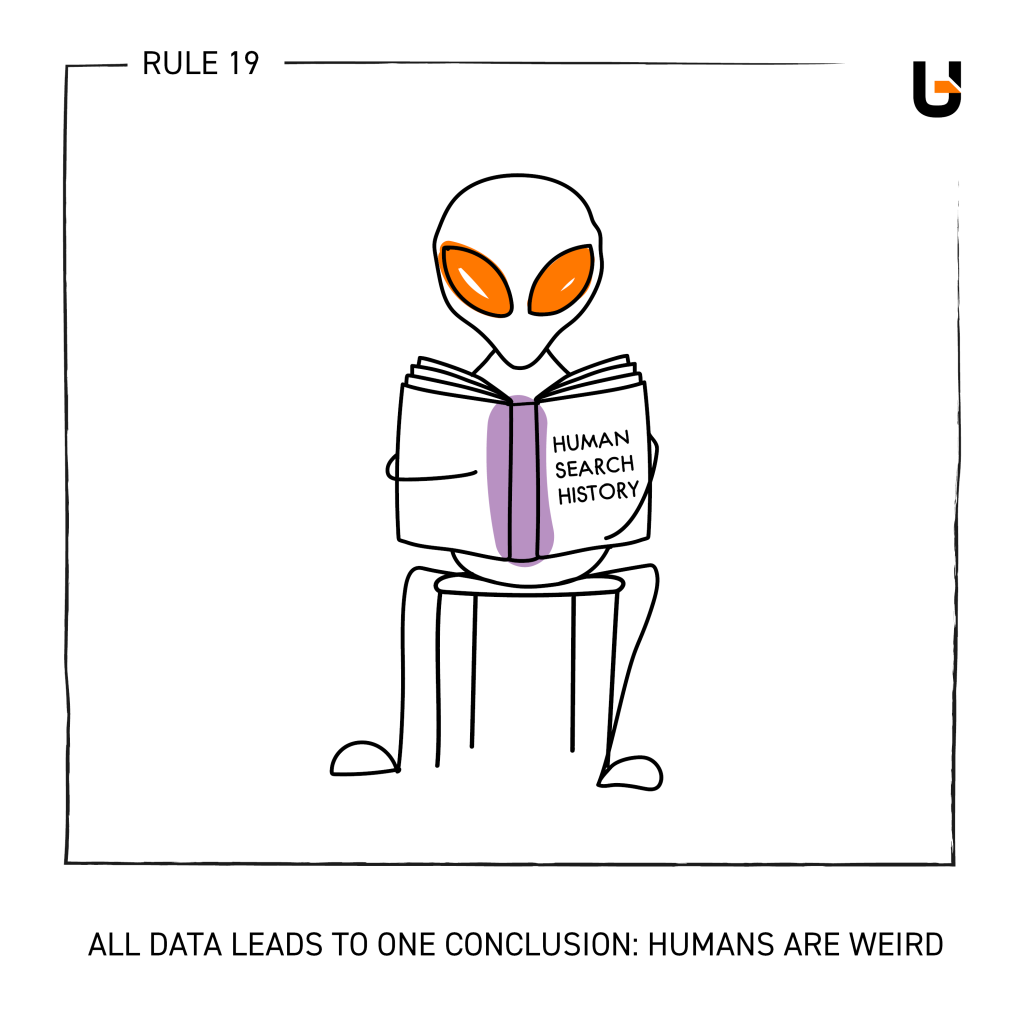
When Does Technology Become Artificial Intelligence?
To be considered artificial intelligence, a system should be able to perform tasks that traditionally require human intelligence, such as reasoning, learning, problem-solving, pattern recognition, natural language processing, and decision-making.
One of the capabilities of both artificial and human intelligence is learning. Learning in the context of AI refers to a system’s ability to improve its skills and performance based on experience and analysis of dependencies in large datasets. This process is fundamental to creating AI systems capable of adapting to new tasks and situations.
All AI models do this – if it can’t adapt based on data, then it simply isn’t artificial intelligence. While there are different types, ranging from simple AI models to fully fledged deep neural networks, they all have to meet this initial criteria.
Types of Machine Learning Algorithms
Artificial Intelligence uses various types of machine learning algorithms to create advanced, intelligent systems:
- Machine Learning (ML): this technique allows computer systems to learn from data without being explicitly programmed. ML algorithms analyze input data, identify patterns, and use this information to make predictions or decisions. Examples include image classification, sentiment analysis in texts, and demand forecasting.
- Neural Networks: these are mathematical models inspired by the biological structures of the brain, used for pattern recognition and information processing. Deep neural networks consist of layers of neurons interconnected and processing input data through different levels of abstraction. They are crucial in many AI systems, especially in tasks related to image and sound processing.
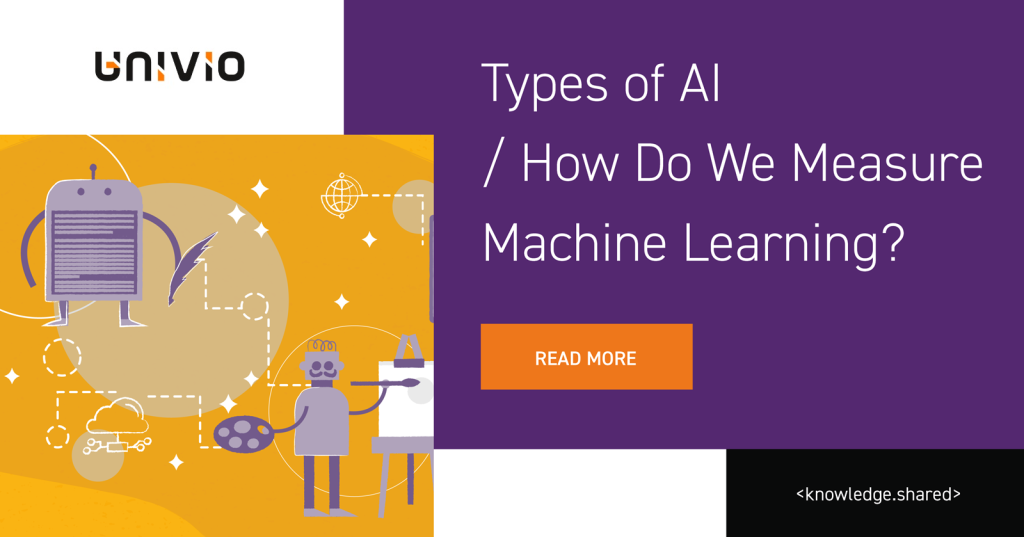
- Deep Learning (DL): a subfield of machine learning that uses multi-layered neural networks to analyze data. Deep learning is particularly effective in processing large amounts of unstructured data, such as images, sounds, and texts. Applications include speech recognition, language translation, image generation, and medical diagnostics.
- Robotics: this field involves designing, constructing, and operating robots. In the context of AI, robotics often uses machine learning algorithms for navigation, object manipulation, and interaction with the environment. Intelligent robots can operate in dynamic and unpredictable environments, making autonomous decisions.
How Does Machine Learning Work?
Machine learning focuses on creating algorithms and computer models capable of independently learning and making decisions based on data. The learning process through experience and drawing conclusions resembles how humans learn. Instead of programming a computer to perform specific tasks, machine learning allows machines to discover patterns and dependencies in data and use them to predict outcomes or make decisions. Properly designed machine learning algorithms are responsible for this.
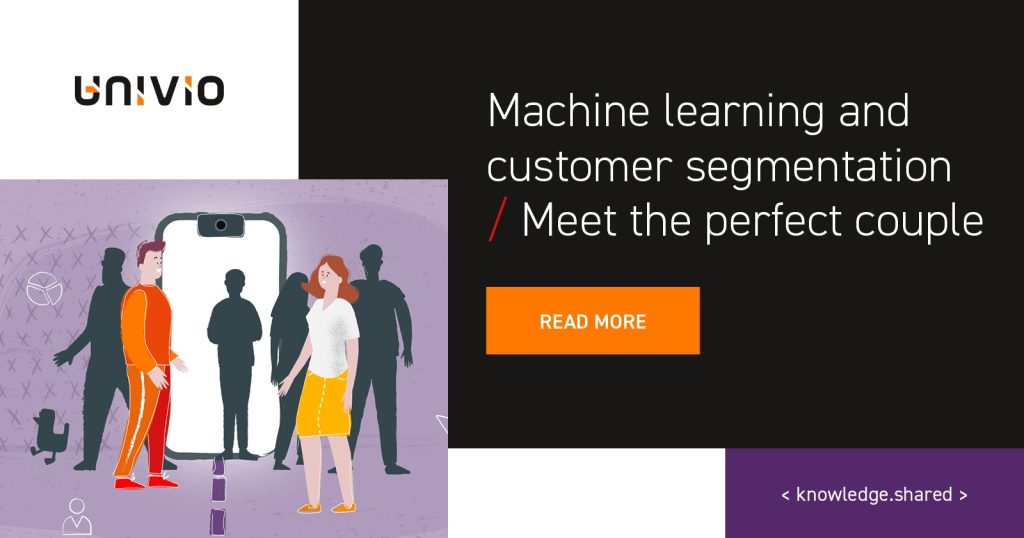
The Process of AI Learning
Imagine a machine that starts as a clean slate and, step by step, learns to recognize patterns, make decisions, and predict future events, similar to how a human does. The AI learning process typically includes several key steps:
- Data Collection and Preparation: data is the foundation of any machine learning model. Collecting relevant data is the first step, often requiring a significant amount of time and resources. The data must then be processed and cleaned to be suitable for training the model.
- Model and Algorithm Selection: the next step is choosing the appropriate model and algorithm to use for learning. The choice depends on the type of problem, available data, and the expected performance of the model.
- Model Training: training the model involves feeding it training data and adjusting its parameters to minimize prediction error. This process can be iterative and requires working with large datasets.
- Validation and Testing: after initial training, the model needs to be tested on separate datasets to ensure it works correctly on previously unseen data. This helps identify issues such as overfitting or underfitting.
- Implementation and Monitoring: the final model is implemented in a real-world environment, where it is used for predictions or decision-making. The model must be monitored and regularly updated as data changes.
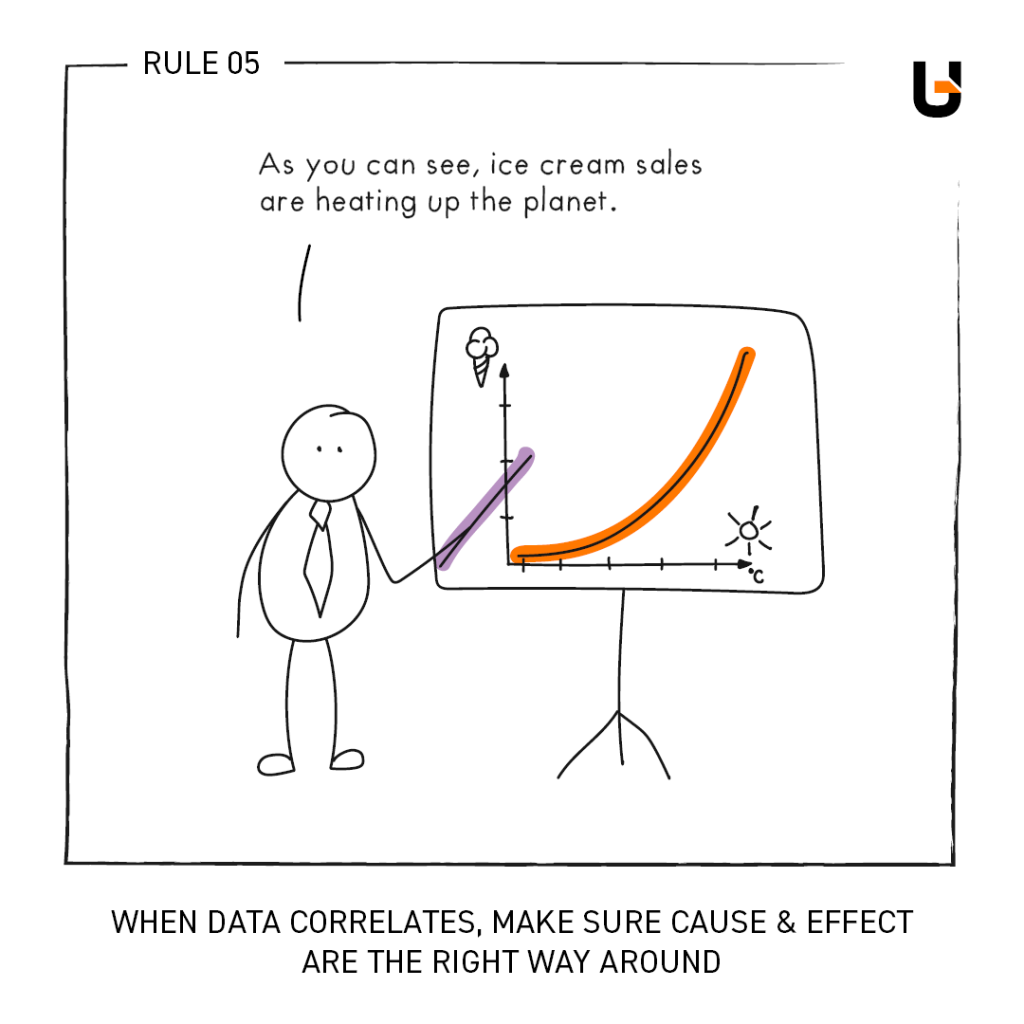
Machine Learning Methods
To effectively solve diverse problems, machine learning uses various methods and techniques. There are three main types of machine learning:
- Supervised Learning: in supervised learning, the model is trained on labeled data, meaning each example in the training set includes both input features and the corresponding output label. The goal is to teach the model how to predict labels for new, unseen data. Typical applications include:
- Classification: assigning labels to categories, e.g., recognizing objects in images.
- Regression: predicting continuous values, e.g., forecasting house prices.
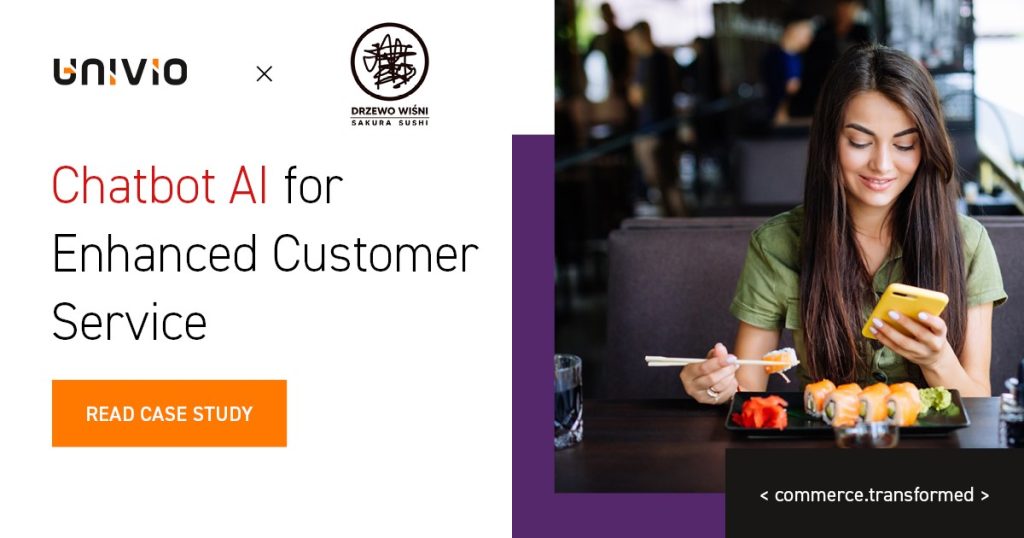
- Unsupervised Learning: in unsupervised learning, the model receives only input data without labels. The goal is to discover the structure or patterns in the data. Common techniques include:
- Clustering: grouping similar data, e.g., customer segmentation.
- Association: discovering associative rules between data, e.g., market basket analysis in retail.
- Reinforcement Learning: in reinforcement learning, an agent (computer program) learns how to act in an environment by interacting with it and receiving rewards for good actions. Through repeated trial and error, the agent optimizes its behavior to achieve the best outcomes. The process is similar to training a dog to perform tricks to receive treats. Applications include:
- Games: algorithms like AlphaGo that learn game strategies.
- Robotics: teaching robots to perform complex tasks.
Challenges of AI Learning
Data is the foundation on which machine learning is based. The quality, accuracy, and completeness of data are crucial for training AI model. It is essential to remember that machine learning models have limitations in distinguishing malicious input data from non-malicious and in identifying incorrect data.
Models are often powered by training data from public, massive databases accessible to outsiders. Such sets can easily become targets for cyberattacks, especially if they are public and free. Over time, malicious data of questionable quality can be considered correct if its formatting remains unchanged.
Given the increasing use of artificial intelligence and machine learning to support critical decisions, e.g., in medicine, the consequences of erroneous decisions can be severe or even tragic. The inability to provide evidence supporting AI-made decisions becomes a barrier both in court and in the public eye. In the business context, such decisions can expose a company to reputational risk, financial losses, and legal problems.
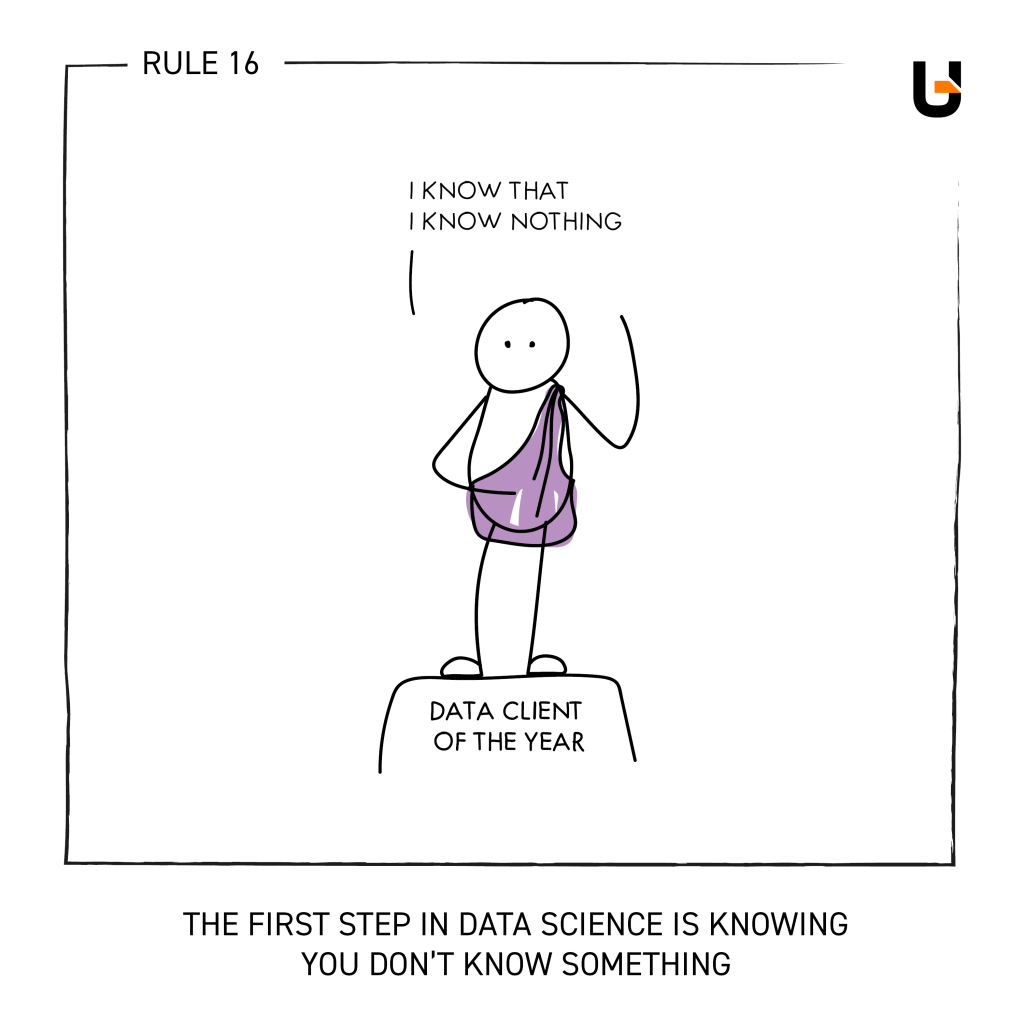
Understanding Training Data
Whether AI training is supervised or unsupervised, training data is an essential component. The quality and availability of such training data – and its continued expansion and updating – plays a key role in all artificial intelligence systems.
Advanced AI models can add their own rsults to the training data, but this typically require a human-in-the-loop. In other words, there needs to be a system for the AI system to know when something is or is not successful.
In this way, the original training data serves as the foundation for your AI training. It will form the benchmark for your AI model’s first successes and learning curve. Over time, however, more recent results will influence its behavior to optimize success rates.
But all of that isn’t to say that you can skimp on training data. In fact, you need to have as much data as possible, for a variety of reasons, namely:
- Remove human bias: Humans are not perfect and everyone does things a little differently. The more data you have, the more such bias can be removed. It’s a basic rule of statistics: the more data poinst you have, the better the AI system can draw the correct correlations and conclusions.
- Cater to outliers: Whilst correlations matter, outliers are still going to occur. Depending on how you’re applying your artificial intelligence, large training data sets will help cater to these wider potential needs.
- Give the AI some freedom: When it comes to model training in unsupervised learning, you need to enable the AI to make the appropriate decisions. The quality of training data and defined goals go a great way towards helping this.
Data Security Methods
Securing data and models is essential to protect the confidentiality of information and ensure the integrity of decision-making processes and the accurate solving of complex problems. There are several ways to achieve this when it comes to artificial intelligence:
- Data Encryption: all data, both stored and in transit, should be encrypted. Encryption ensures that even if data is intercepted by unauthorized persons, it will be unreadable without the proper decryption keys.
- Identity and Access Management (IAM): IAM systems allow controlling who has access to data and models and determining the level of access. Users should have access only to the data and functions necessary to perform their duties.
- Regular Audits and Monitoring: conducting regular security audits and monitoring activities in systems in real-time allows early detection and response to suspicious actions. Audits also help identify weaknesses in the security system.
- Protection Against External and Internal Attacks: companies must be prepared for attacks both from outside (hackers) and from within (disloyal employees). Protection should include firewalls, intrusion detection systems (IDS), intrusion prevention systems (IPS), and antivirus software.
- Secure Storage of AI Models: AI models, especially high-value ones, should be stored in secure environments with controlled access. This may include secure servers, clouds with advanced security features, or isolated systems.
- Employee Training: staff should be regularly trained on data security and best practices. Knowledge of potential threats and ways to avoid them is crucial for maintaining the security of the entire organization.
- Incident Management: a company should have a plan for responding to security incidents. This plan should include procedures for identifying, assessing, countering, and reporting incidents. Quick and effective response can significantly reduce the impact of a security breach.
- Secure Data Sharing: when data needs to be shared with external partners or clients, it is essential to use secure sharing methods, such as secure portals, data tokenization (replacing sensitive data with non-sensitive data), or temporary access keys.
Artificial Intelligence: More Than Just Training Data
In summary, AI training process is complex, and involves data collection and processing, model selection, training, validation, and implementation. Despite the challenges this field presents, the future prospects are incredibly promising, making it one of the most important and exciting areas of modern science and technology.